Image Resolution and GSD: What can be considered good enough?
Good enough is a relative term that is hard to quantify. It basically comes down to what is good enough for you and your teams in terms of detail.
While most objects of interest might be easy to identify, it’s the smallest details that determine what is good enough.
It starts with knowing what type of components and defects must be recognizable in the images (whether by human or AI) and how small they are expected to be. Remember to consider future requirements as the data you collect today will provide a head start when you expand to detecting other defects — you will already have a training data set.
Once you know the defects that must be recognizable, you need to choose a camera that can capture the required image resolution or Ground Sampling Distance (GSD) at the required safety distance for what you are looking to identify.
As you can see in the examples below, GSD makes a difference to the identification of defects in images, which in turn impacts the confidence of the identification.
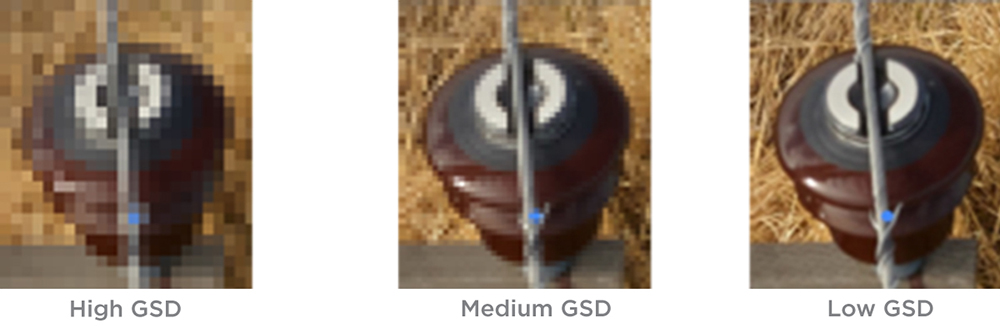
It is important to note that it is impossible to see anything smaller than one pixel and typically several pixels across are needed for sufficient confidence to make Operations and Maintenance decisions. O&M refers to the activities required to run, manage, and sustain the functionality and performance of systems, equipment, and infrastructure.
For example, imagine you need to detect a 2-millimeter-wide crack. A GSD of 2 mm would only give you one pixel across the crack, which would not be sufficient. On the other hand, a 0.2 mm GSD would give you 10 pixels across, which would be enough for a human or AI analysis.
Typically, a low GSD is achieved either by flying closer to the asset or by using a zoom lens. But both significantly reduce coverage, negatively impacting efficiency. Flying closer also dramatically increases operational risks.
One other dimension of “good enough” concerns what you want to do with the image data you’ve captured going forward. You must consider if AI is a part of your future operational excellence. Given its current prevalence, it is hard to imagine a future without it.
As you and your inspection service provider determine what meets your future needs, remember the ‘garbage in, garbage out’ principle.
Successful AI relies on ample high-quality data for effective training but also on a collaborative effort, combining the strengths of AI training over time with the invaluable human touch. Even as we embrace virtual manual inspections conducted by our inspectors, it is essential to anticipate that what suffices today may fall short tomorrow. Therefore, envision future requirements when defining ‘good enough.’ Commence building a superior dataset, blending AI insights with human expertise early on, to expedite your organization’s journey towards harnessing the full potential of advanced AI capabilities.
Ground Sampling Distance explained
Ground Sampling Distance (GSD) describes the size of an image’s pixels. The smaller the pixels (and thereby the GSD), the smaller the details that are recognizable on the asset of interest.
You need to match your GSD to what you need to see in the image.
In both human and AI analyses, an insufficient GSD is one of the most common causes of false positives and missed defects.
Key factors to consider when choosing a camera sensor
It is tempting to only consider resolution because it is easy to quantify and compare. However, sharpness and optical quality are crucial to get the full benefit of a camera sensor’s resolution.
Regardless of how you analyze your data — human, AI, or a blend of both — if it is not of a high enough quality, small details at a pixel level will not be reliably identifiable and critical defects might be missed. This impacts productivity.
Quality is everything
High quality images are a prerequisite for high end-to-end productivity.
Remember to consider the desired level of confidence. The lower the requirements, the less it will demand of the image quality.
Optical quality must be good across the entire image area. You need a camera sensor with a good dynamic range to capture images that contain sufficient detail in both bright and dark regions as pilots operate in suboptimal lighting conditions.
Imagine you are conducting an inspection of a cell tower on a bright sunny day. Data captured in natural light and direct sunlight produce highlights and deep shadows. The large camera sensor must be able to handle this range within a single image. If not, productivity, logistics, and detection quality can be significantly reduced.
Below you can see an example of quality:
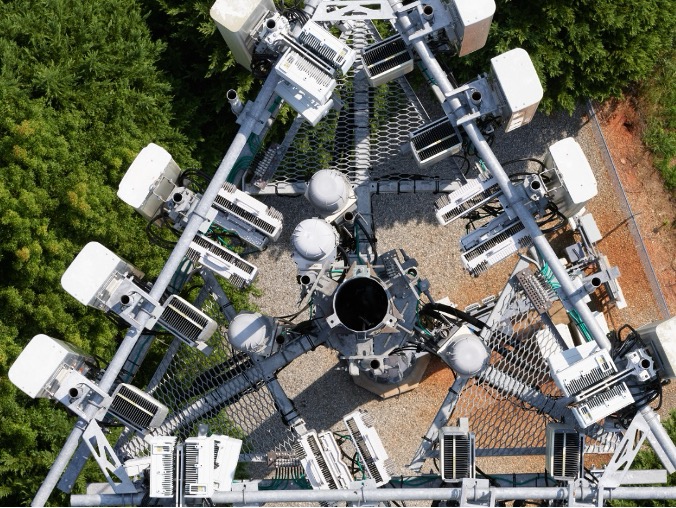
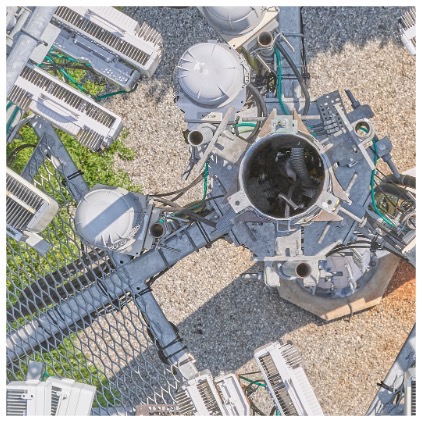
Achieving optimal GSD and maximizing efficiency
Coverage and Image Size explained
Coverage is dictated by image size, or more precisely, by the number of pixels in an image and not by the image’s dimensions.
The larger the image size (pixels), the larger the area that can be covered by a single image at the required GSD.
Maybe you are getting a good GSD by flying close to the asset or zooming-in on it with a standard camera, but you have noticed this takes much longer and requires a lot of images, making it inefficient.
However, you can achieve an optimal GSD without sacrificing efficiency by using high-resolution cameras.
Because their sensors have more pixels, the number of pixels on the asset is significantly higher.
While a standard camera might have to fly as close as 3 m to the asset and take a lot of images, a high-resolution camera could be as far away as 15 m with the same GSD, so far fewer images are required.
Fewer images means that the mission can be executed simpler and faster.
Key takeaways when scoping requirements
- Scope your inspection requirements by defining your GSD requirements based on the defect you need to identify.
- Expect your service provider to discuss this with you because making the right choice is crucial.
If you are inspecting a large, complex asset with a low GSD but with a standard camera, the number of pixels (the image size) in each image will be small. In this case, you will need a lot of images, which will not only reduce data capture efficiency, but also the efficiency of other activities, such as image transfer, annotation, and visualization.
The result? Reduced end-to-end productivity.
More megapixels, fewer shots: optimizing image count for optimal GSD
Example object: H: 70 m B: 25 m | |||
1 mm GSD | 2 mm GSD | 5 mm GSD | |
20 MP with Zoom | Distance: 1 to 20m
Frame size: 5 x 4 m # Images: 68 |
Distance: 2 to 40 m
Frame size: 10 x 8 m # Images: 38 |
Distance: 5 to 100 m
Frame size: 26 x 19 m # Images: 14 |
45 MP with 35 mm Lens | Distance: 8 m
Frame size: 8 x 5 m # Images: 54 |
Distance: 16 m
Frame size: 16 x 10 m # Images: 28 |
Distance: 40 m
Frame size: 41 x 27 m # Images: 6 |
100 MP with 80 mm Lens | Distance: 21 m
Frame size: 12 x 9 m # Images: 30 |
Distance: 42 m
Frame size: 23 x 18 m # Images: 22 |
Distance: 106 m
Frame size: 44 x 58 m # Images: 4 |
Examples of how image size, lens, and distance impacts to number of images required to cover a certain area with a certain GSD
Key takeaways when choosing a camera sensor
- Focus on the GSD requirements of the smallest object that needs to be identified.
- Make sure you include future requirements for your data and consider what role AI will have. High quality AI analysis requires high quality training data.
- Consider your options for achieving the required GSD. Is it possible to fly close enough to the object to get the required GSD or do I need to use a longer lens?
- Consider the trade-off between sensor cost and cost of operation. Smaller sensors will require more images, which will increase your mission time and post-mission analysis.
- Higher resolution does not mean larger projects. Remember to compare project sizes rather than file sizes.
A note on data volume
It might be logical to assume capturing, for example, 100 Mega Pixel images would result in a huge amount of data compared to capturing 20 Mega Pixels.
This is not the case.
With a lower resolution camera, you get individual images with a smaller number of pixels, so you need more overlapping images to ensure all data is captured.
With a higher resolution camera, individual images have more pixels, so fewer images are needed.
As a result, the resolution of the camera does not impact the amount of data captured. What truly dictates the amount of data captured is the GSD needed and the size of the asset.
Share this on social media: